Data annotation plays a major role in the training of machine learning models, particularly in supervised learning, which involves labeled data for training the model. Infosearch BPO Services offers 16 data annotation services for machine learning, computer vision, autonomous vehicles, robotics, agriculture, healthcare, and more. Contact us to outsource your annotation support.
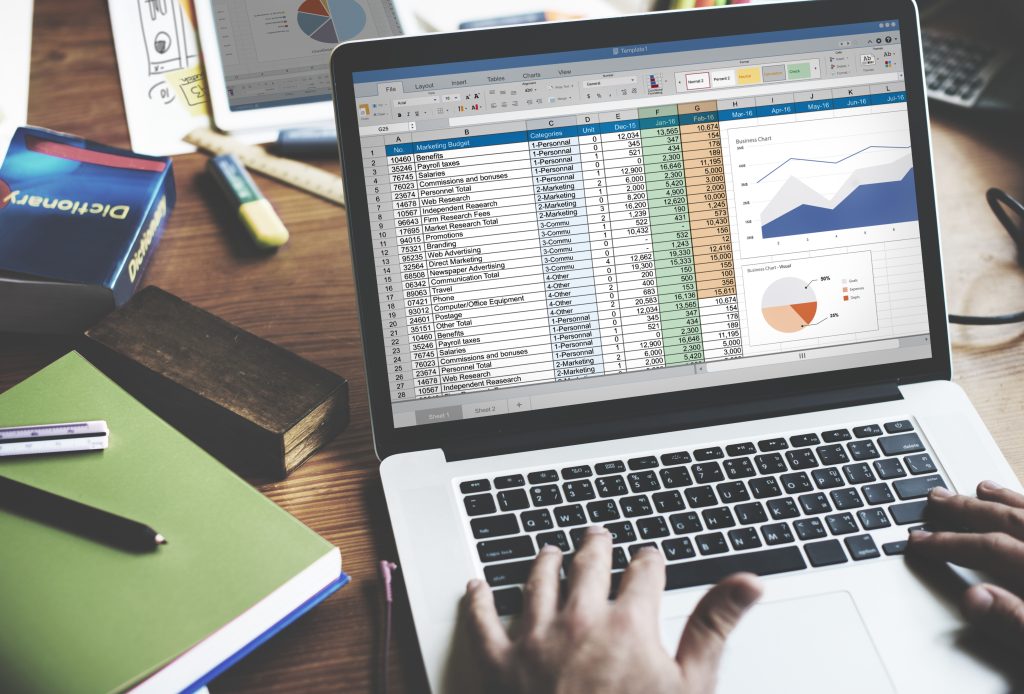
Here are some best practices for data annotation:
1. Define clear annotation guidelines:
It is important to brief the annotators on the guidelines in order to achieve consistency with annotations.
We will have to provide examples and edge cases to the annotators
2. Train annotators:
Hold training sessions for annotators to make sure that they understand the guidelines and are able to apply them correctly.
Carry out periodical refresher exercises for uniformity of outcomes over time.
3. Quality Control:
Strengthen the system of quality control to ensure that all the annotations are accurate.
Consider using a subsample for inter-annotator agreement by having multiple annotators label the same data separately and check for reliability.
4. Iterative Feedback:
Develop a dialogue cycle between annotators and data scientists by answering questions with hands-on experience and revisions of existing recommendations.
5. Use multiple annotators:
Use multiple annotators as often as possible in order to attain high reliability while minimizing individual biases.
6. Handle Ambiguity:
Specifically, provide clear indications of how to approach ambiguity within the annotation guidelines.
Allow annotators to flag doubtful cases for re-checking.
7. Use consistent terminology:
Ensure that the same terminology is used by all annotators across different annotation projects.
Explain specific technical terms in a glossary.
8. Use specialized tools:
Use of annotation tools developed for your specific kind of data (text, images, audio, etc.)
Look out for those with work-sharing abilities and track.
9. Balance Speed and Accuracy:
Strike a balance between the rate of annotation and the quality of annotations. Quality matters more than fast annotations.
10. Handle Imbalanced Classes:
Make sure you are conscious of class imbalances in your datasets so that annotations that could lead to biases when model training is done can be avoided.
11. Protect privacy:
When dealing with sensitive information, consider using approaches to guarantee privacy, including anonymization or suitable aggregation of data.
12. Document Changes:
Log any changes or updates to the annotation guidelines in order to remain transparent so that you may go back to these guidelines at any time in the future.
13. Scalability:
Develop scalable design annotation processes as the data becomes larger. Where appropriate, use automation or semi-automation.
14. Regular Audits:
Regularly audit annotated data, correcting errors as necessary to maintain consistent quality over time.
15. Legal and Ethical Considerations:
This becomes more critical where it involves sensitive data, especially when dealing with miners’ medical reports.
Adhering to these best practices will enable you to create annotations with high quality and integrity that can support reliable machine learning models. We at Infosearch, follow all that mentioned above and provide you quality annotation services.
How to contact Infosearch:
Website: www.infosearchbpo.com Email: enquiries(at)infosearchbpo(dot)com
Recent Comments