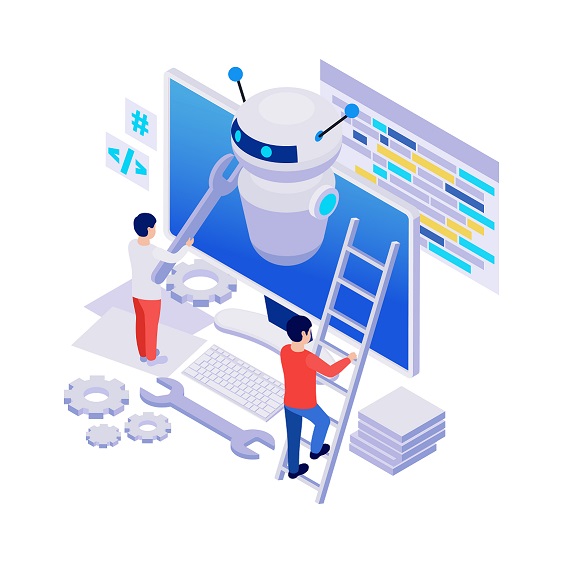
Introduction
Are you looking to outsource data labelling services? If yes, then this article is for you. Whether you start as a small business or a large corporation, this guideline created for you will be all that you need to make a wise choice based on the facts given.
Infosearch BPO is a global outsourcing company that specializes in data annotation and labeling services. Infosearch understands the importance of data labelling in machine learning and so provides accurate, error-free annotated datasets for your business.
Human labelling of data is a must for the development of machine learning and AI, but it is necessary, time-consuming, and resource-demanding. Outsourcing gets covered right in that place. Leveraging the ability of third-party data providers can allow you to save the precious time and resources of your company, as well as validate quality in a sense.
Through this document, we will dive into the advantages of data labelling outsourcing, the critical issues one should be concerned with when opting for a provider, and the best approaches for establishing a successful partnership. In addition, we will uncover the various categories of data labelling services, which encompass video and image annotation, speech and text recognition, etc.
The Role of Data Labeling in Machine Learning:
Data annotation is important for machine learning and AI technology. It encompasses labeling raw data by means of adding specific tags or labels with the purpose of creating labeled data set, which in turn may be used for training machine learning systems. The effect on the well-built labels for the data can be both performance and the accuracy of the trained models.
Moreover, accurate data labelling is essential for all the purposes mentioned above: image and object recognition, natural language processing, sentiment analysis, and speech perception. Data labelling is the process of labelling or grouping data into categories. Consequently, without proper labelling, machine learning algorithms can’t process and interpret patterns and relationships of the data.
Also, supervised learning offers a viable option to deliver accurate results in unlabeled examples, since data labels are used as the example to which they are compared. This may also be achieved through the process of iterative training and testing, enforcing the accuracy and dependability of the AI models employed. However, the extent to which we will reap benefits from the machine learning efforts depends on the quality of data labelling. So we should go for high-end data labelling to make the machine learning project a success.
Challenges of Data Labelling and the Need for Outsourcing
There is no doubt that labelling data is as important as the data itself and without it, machine learning algorithms won’t be able to reach their full potential. The only problem is that it can be a time-consuming process and requires a lot of resources. Manual annotations of a big dataset usually eat up a lot of human time, attention, and involvement in domains’ affairs. This can provide tough challenges to companies that don’t have the power or potential; or have fewer internal capabilities.
Besides, domain-specific knowledge for proper annotations is without a doubt an important requirement as well. Depending on what kind of data is used, there could be different labeling techniques, for example, bounding boxes, polygons, keypoints and semantic segmentation, among others. When you dedicate an in-house team to be trained and maintained, you have to deal with the costs of various expenses and the time-consuming process, which is itself both expensive and time-consuming.
Data outsourcing overcomes these problems by offering an opportunity for companies to benefit from the knowledge on a super-specialized level. For this purpose, these companies have expert-annotated groups that have mastered the labelling techniques and can handle a large quantity of information at high speed. Through data labelling, firms can actually save time, cut costs and get annotations done that are precise enough.
Benefits of Data Labelling Outsourcing
Outsourcing data labelling is evidently of great advantage to companies of any size. Here are some key advantages:
1. Time and Cost Savings
With data labelling outsourcing, companies can free up precious time, space, and money. Rather than wasting your vital hours or days trying to handle the data labeling process, you can concentrate on main business operations, and then rely the service provider to take care of labelling services. Hence, you can advance your AI projects to get them into the market in a much shorter time frame.
Data labelling through outsourcing also saves you money. Hiring and training your own in-house team may take a big chunk of your finances, especially if you need a team with all the skill levels you need to deliver the services at hand. Outsourcing eliminates having to do the recruitment, training, and infrastructure expenditures. In their place, these resources and efforts can be directed into more valuable programs.
2. Access to Expertise
The core specialty of the outsourcing data labelling services providers is annotation processes such as object detection and image segmentation, with the company having a team of dedicated annotators to this regard. They are the ones who have skill and technicality to compete with the wide awareness of media, images, videos, text, and audio. Since the outsourcing service provides with expert knowledge, you are in a strong position to correct and update the information annotated.
3. Scalability and Flexibility
Database labelling outsourcing is scalable and gives a client a choice to pick what they want. Your outsourcing partnership would remain able to cater to your needs of growing data labelling, You are allowed to scale up your partnership to tackle larger volumes of data. This freedom makes you able to follow the project modifications and prevents you from clogging the AI development process.
4. Quality Control
These data labelling outsourcing companies would have established quality control processes to maintain a high level of labelling accuracy and consistency. They use top-notch quality assurance systems by employing such practices as annotation tripling, conducting quality audits regularly, and feedbacking system development. It will therefore maintain strict quality, ensure labeling accuracy and provide a dataset of high quality.
5. Focus on Core Competencies
Using the techniques of data labeling, outsourcing gives the company chance to focus on its competent areas. There would be more time and space for the companies to produce innovative models of AI for marketing, customer experience improvement and business growth since non-core activities, like data annotation, would be outside of their focus.
Outsource your data annotation and labelling services to Infosearch. Contact us for more details.
Recent Comments